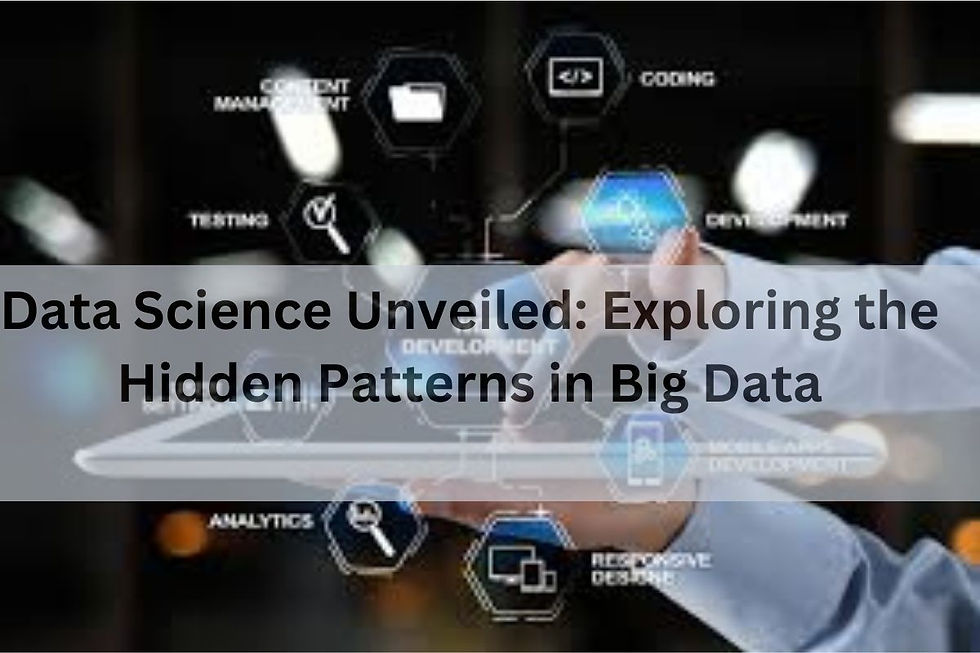
In the digital age, we are constantly inundated with vast amounts of data from various sources. From social media interactions to online shopping habits, from sensor data in smart cities to medical records, the volume of data generated daily is staggering. Amidst this data deluge lies valuable insights and patterns waiting to be uncovered. This is where the field of data science comes into play, offering tools and techniques to extract meaningful information from big data.
Unraveling the Mysteries of Big Data
Big data refers to datasets that are so large and complex that traditional data processing methods are inadequate to deal with them. These datasets come in various forms, structured and unstructured, and present significant challenges in terms of storage, analysis, and interpretation. However, within these vast troves of data lie hidden patterns, trends, and correlations that can offer valuable insights for businesses, governments, and researchers alike.
The Rise of Data Science
Data science is the interdisciplinary field that combines statistical analysis, machine learning, data visualization, and computer science to extract knowledge and insights from data. It encompasses a range of techniques and methodologies aimed at uncovering patterns, making predictions, and informing decision-making processes. From predictive analytics to natural language processing, data science techniques are deployed across industries to solve complex problems and drive innovation.
Extracting Meaning from the Noise
One of the primary challenges in working with big data is separating signal from noise. With vast amounts of data comes a significant amount of irrelevant or redundant information. Data scientists employ various techniques such as data cleaning, preprocessing, and feature selection to eliminate noise and focus on the most relevant aspects of the data. This process lays the groundwork for further analysis and interpretation.
Unsupervised Learning: Finding Hidden Structures
Unsupervised learning algorithms play a crucial role in uncovering hidden patterns within big data. These algorithms operate without labeled data and aim to identify underlying structures or relationships within the dataset. Clustering algorithms, such as k-means clustering and hierarchical clustering, group similar data points together, revealing natural groupings or clusters within the data. Dimensionality reduction techniques, such as principal component analysis (PCA) and t-distributed stochastic neighbor embedding (t-SNE), help in visualizing and understanding high-dimensional data by projecting it onto lower-dimensional spaces.
Supervised Learning: Making Predictions
Supervised learning algorithms, on the other hand, learn from labeled data to make predictions or classifications. These algorithms are trained on historical data with known outcomes, enabling them to generalize patterns and make predictions on unseen data. Techniques such as regression analysis, decision trees, support vector machines (SVM), and neural networks are commonly used in supervised learning tasks. Whether it's predicting customer churn, detecting fraudulent transactions, or diagnosing diseases, supervised learning algorithms enable organizations to make data-driven decisions and take proactive measures.
The Power of Visualization
Data visualization plays a crucial role in data science by providing intuitive representations of complex datasets. Visualization techniques such as scatter plots, histograms, heatmaps, and network graphs help in exploring patterns, identifying outliers, and communicating insights effectively. Interactive visualization tools allow users to interact with data dynamically, gaining deeper insights and facilitating exploratory analysis. Visualization not only aids in understanding data but also in communicating findings to stakeholders in a clear and compelling manner.
Ethical Considerations and Privacy Concerns
As data science continues to revolutionize industries and transform societies, it also raises important ethical considerations and privacy concerns. The collection and analysis of large-scale datasets raise questions about consent, data ownership, transparency, and fairness. Data scientists must adhere to ethical guidelines and regulations to ensure responsible and ethical use of data. By prioritizing privacy protection, data anonymization, and informed consent, organizations can build trust with users and stakeholders while leveraging the power of data science for societal good.
Embracing the Challenges of Data Science
Despite the remarkable advancements in data science, challenges persist in harnessing the full potential of big data. One of the ongoing challenges is the sheer volume and velocity of data generated, requiring scalable infrastructure and efficient algorithms for processing and analysis. Additionally, ensuring data quality and integrity remains a paramount concern, as inaccuracies or biases in the data can lead to erroneous conclusions and decisions.
Moreover, the interdisciplinary nature of data science requires collaboration across domains, including mathematics, computer science, domain expertise, and ethics. Effective communication between data scientists, domain experts, and stakeholders is essential for framing the right questions, interpreting results, and translating insights into actionable strategies. By fostering a culture of collaboration and continuous learning, organizations can leverage diverse perspectives and expertise to tackle complex problems and drive innovation.
Empowering Decision-Making with Data
At its core, data science empowers organizations to make informed decisions based on evidence and insights derived from data. Whether it's optimizing business processes, personalizing user experiences, or improving public services, data-driven decision-making enables organizations to stay competitive, agile, and responsive to changing environments. By leveraging predictive analytics, organizations can anticipate trends, identify opportunities, and mitigate risks, gaining a strategic edge in the marketplace.
Furthermore, data science has the potential to drive social impact by addressing pressing challenges such as healthcare disparities, environmental sustainability, and social inequality. By analyzing healthcare data, for example, data scientists can identify patterns of disease prevalence, optimize treatment protocols, and improve healthcare outcomes for populations around the world. Similarly, by analyzing environmental data, researchers can monitor and mitigate the impact of climate change, preserving ecosystems and safeguarding the planet for future generations.
Cultivating a Data-Driven Culture
As organizations recognize the transformative power of data science, there is a growing emphasis on cultivating a data-driven culture across all levels of the organization. This involves not only investing in technology and infrastructure but also nurturing talent, fostering data literacy, and promoting data-driven decision-making as a core organizational value. By empowering employees with the skills and tools to access, analyze, and interpret data, organizations can democratize data and foster innovation at scale.
Moreover, leadership plays a crucial role in championing data-driven initiatives, setting clear objectives, and aligning data science efforts with strategic priorities. By fostering a culture of experimentation and learning, leaders can encourage risk-taking, iteration, and continuous improvement, enabling organizations to adapt and thrive in an increasingly data-centric world.
Looking Ahead: The Future of Data Science
As we look to the future, the role of data science will continue to evolve in response to emerging technologies, societal trends, and global challenges. With the advent of artificial intelligence, the Internet of Things, and edge computing, data scientists will have access to new sources of data and advanced analytics capabilities, enabling them to tackle complex problems with greater precision and efficiency.
Furthermore, as data privacy regulations evolve and ethical considerations gain prominence, data scientists will need to prioritize responsible data stewardship and transparency in their practices. By embracing principles of fairness, accountability, and transparency, data scientists can build trust with users, stakeholders, and society at large, fostering a culture of ethical innovation and responsible AI.
In conclusion, data science holds the promise of unlocking hidden insights, driving innovation, and shaping the future of our digital world. By embracing the challenges, empowering decision-making, cultivating a data-driven culture, and prioritizing ethics, organizations can harness the full potential of data science to create positive impact and build a more inclusive and sustainable future for all.
Conclusion:
In conclusion, data science presents an unparalleled opportunity for innovation and growth in Ahmedabad's digital landscape. Leveraging advanced analytics, machine learning, and visualization, data scientists can unearth crucial insights, empower decision-making, and address multifaceted challenges across sectors. As Ahmedabad navigates the complexities of big data, it's imperative to uphold ethical standards, foster collaboration, and nurture a culture of data-driven innovation. By embracing these principles, Ahmedabad can harness the full potential of data science to drive positive change, sustainable development, and a brighter future for generations to come. Additionally, for those seeking to deepen their expertise, numerous Data science courses in Ahmedabad, Nashik, Delhi and other cities in India cater to diverse skill levels, providing the necessary tools and knowledge to excel in this burgeoning field and contribute meaningfully to its advancement.
Comments