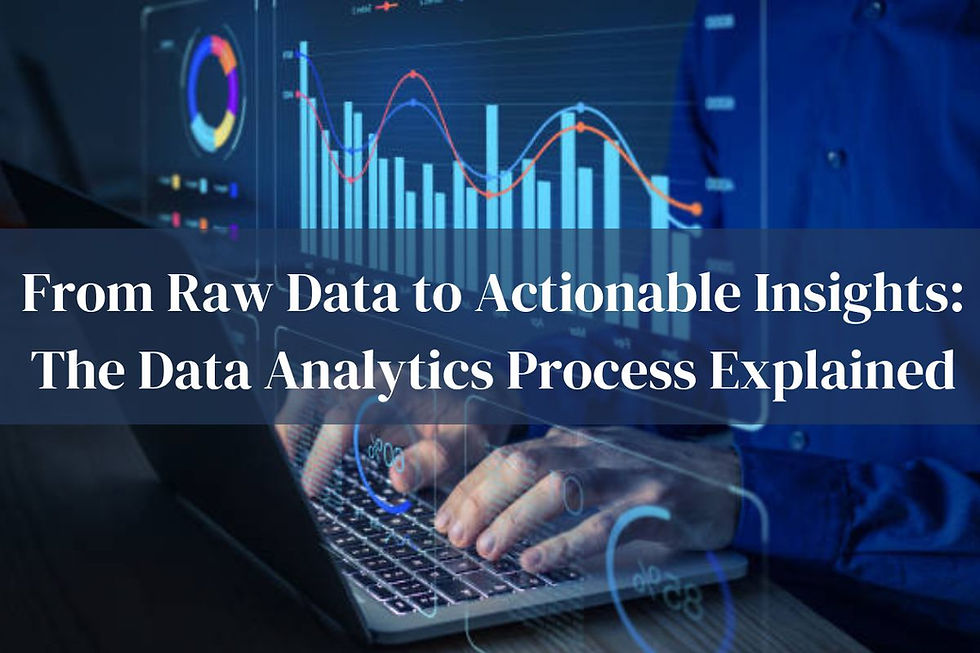
Introduction
In today's data-driven world, organizations are sitting on a goldmine of information. But this raw data is useless unless it's transformed into actionable insights that can inform better decision-making. This is where data analytics comes in.
Data analytics is the process of extracting meaningful information from data. It's a multi-step process that involves collecting, cleaning, analyzing, and visualizing data to uncover patterns, trends, and relationships. These insights can then be used to solve problems, improve efficiency, and drive business growth.
Here's a breakdown of the key steps in the data analytics process:
1. Define Objectives and Formulate Questions:
The first step is to understand what you're hoping to achieve with your data analysis. What are your business goals? What questions are you trying to answer? Clearly defining your objectives will help you determine the type of data you need to collect and the appropriate analytical techniques to use.
2. Data Collection:
Once you know what you're looking for, you can start gathering data. This data can come from various sources, including internal databases, customer surveys, social media, and web traffic logs. The important thing is to ensure the data is relevant and accurate to your objectives.
3. Data Cleaning and Preprocessing:
Raw data is rarely perfect. It may contain errors, inconsistencies, and missing values. Data cleaning involves identifying and correcting these issues to ensure the accuracy of your analysis. This step may involve removing duplicates, formatting data consistently, and imputing missing values.
4. Exploratory Data Analysis (EDA):
EDA is the initial phase of analysis where you get to know your data. You'll use various techniques like data visualization to identify patterns, trends, and relationships within your dataset. This exploration helps you understand the characteristics of your data and guides you in making informed decisions about further analysis.
5. Model Building and Evaluation (if applicable):
Depending on your objectives, you may want to build a statistical model to predict future outcomes or classify data points. This could involve techniques like machine learning or regression analysis. Once you've built a model, you need to evaluate its performance to ensure it's reliable and accurate.
6. Interpretation and Actionable Insights:
The final step is to interpret the results of your analysis and translate them into actionable insights. What do the findings mean for your business? How can you use this information to improve operations, target marketing campaigns, or develop new products?
7. Communication and Action:
Once you have actionable insights, it's crucial to communicate them effectively to stakeholders who can take action. This may involve creating reports, presentations, or dashboards that clearly showcase the findings and their implications.
By following these steps, you can transform raw data into actionable insights that can drive real business value. Data analytics is a powerful tool, but it's only effective when used strategically and with a clear understanding of the process.
Conclusion
Data analytics is a transformative journey from raw data to actionable insights. By following a structured process, you can unlock the hidden potential within your data and use it to make data-driven decisions that fuel innovation, optimize operations, and achieve your business goals. Remember, data is just the beginning. It's the meaningful stories you extract from it that will truly empower your organization.
If you're interested in learning more about data analytics and transforming your career, there are numerous Data Analytics course providers in Gurgaon, Ahmedabad, Nashik, Delhi and other cities in India offering comprehensive programs to equip you with the necessary skills and knowledge. Consider researching these institutes to find the program that best aligns with your goals and learning style.
Comments