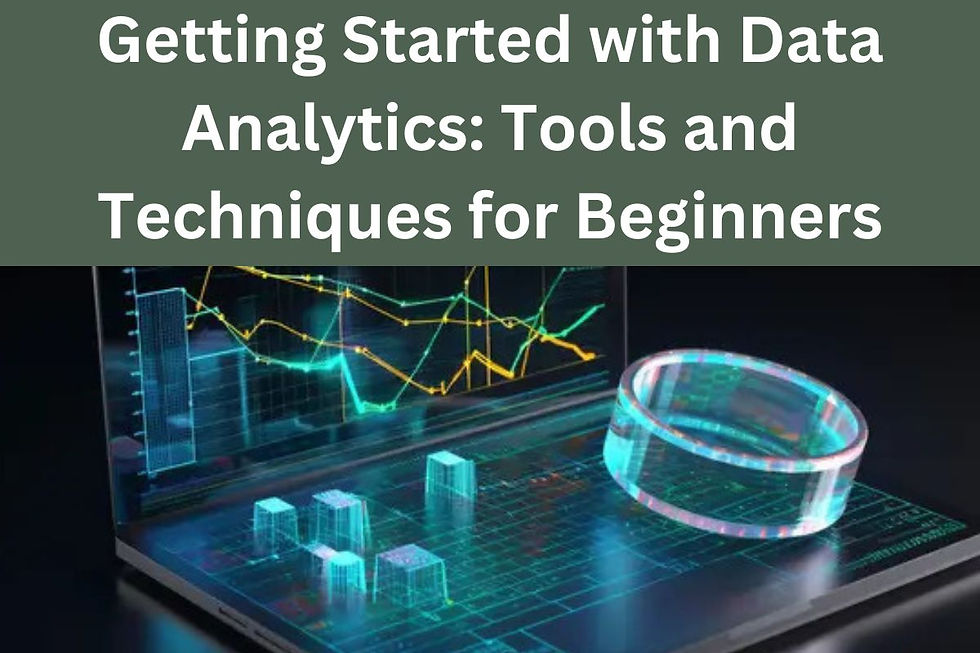
Introduction
Data analytics has become an integral part of modern business strategies. It involves examining raw data to draw conclusions and make informed decisions. The relevance of data analytics spans across various sectors including finance, healthcare, marketing, and more. By leveraging data analytics, organizations can uncover patterns, predict trends, and gain a competitive edge.
Understanding Data Analytics
What is Data Analytics?
Data analytics refers to the process of analyzing raw data to extract meaningful insights. It involves various techniques and tools to examine data sets and derive actionable information.
Importance of Data Analytics
Data analytics helps organizations make data-driven decisions, improve operational efficiency, and enhance customer experiences. It also plays a crucial role in identifying market trends and opportunities.
Types of Data Analytics
Descriptive Analytics
Descriptive analytics focuses on summarizing historical data to understand what has happened. It uses data aggregation and data mining techniques to provide insights into past performance.
Diagnostic Analytics
Diagnostic analytics delves deeper into data to understand the causes of past events. It helps in identifying patterns and correlations to explain why certain outcomes occurred.
Predictive Analytics
Predictive analytics uses statistical models and machine learning techniques to forecast future outcomes. It analyzes historical data to predict trends, behaviors, and events.
Prescriptive Analytics
Prescriptive analytics goes beyond prediction to recommend actions. It uses optimization and simulation algorithms to suggest the best course of action based on the predicted outcomes.
Key Components of Data Analytics
Data Collection
Data collection involves gathering relevant data from various sources. This can include transactional data, social media data, sensor data, and more.
Data Cleaning
Data cleaning is the process of correcting or removing inaccurate records from a data set. It ensures that the data used for analysis is accurate and reliable.
Data Integration
Data integration involves combining data from different sources to provide a unified view. It helps in consolidating data for more comprehensive analysis.
Data Analysis
Data analysis is the core of data analytics. It involves applying statistical and computational techniques to uncover insights from data.
Data Visualization
Data visualization uses graphical representations to present data insights. It makes it easier to understand complex data through charts, graphs, and dashboards.
Popular Data Analytics Tools
Excel
Excel is a widely used tool for data analysis due to its simplicity and versatility. It offers various functions and add-ins for statistical analysis and visualization.
SQL
SQL (Structured Query Language) is essential for managing and querying relational databases. It allows users to retrieve and manipulate data efficiently.
Python
Python is a powerful programming language for data analysis. It has libraries like Pandas, NumPy, and Matplotlib that facilitate data manipulation and visualization.
R
R is a statistical programming language designed for data analysis. It offers a wide range of statistical and graphical techniques.
Tableau
Tableau is a data visualization tool that helps in creating interactive and shareable dashboards. It allows users to explore data visually and uncover insights.
Power BI
Power BI is a business analytics tool by Microsoft. It provides interactive visualizations and business intelligence capabilities with an interface easy enough for end users to create their own reports and dashboards.
Techniques in Data Analytics
Statistical Analysis
Statistical analysis involves collecting and analyzing numerical data to identify trends and patterns. It includes descriptive statistics, inferential statistics, and more.
Machine Learning
Machine learning is a subset of artificial intelligence that uses algorithms to learn from data and make predictions. It includes techniques like regression, classification, clustering, and more.
Data Mining
Data mining involves exploring large data sets to discover patterns and relationships. It uses techniques like association, sequence analysis, and anomaly detection.
Text Analytics
Text analytics involves analyzing text data to extract meaningful information. It includes techniques like sentiment analysis, topic modeling, and text classification.
Time Series Analysis
Time series analysis deals with data that is indexed in time order. It involves techniques like moving averages, exponential smoothing, and ARIMA models.
Applications of Data Analytics
Business Intelligence
Data analytics enhances business intelligence by providing insights that help in decision-making, strategy formulation, and performance monitoring.
Marketing
In marketing, data analytics helps in understanding customer behavior, segmenting audiences, and measuring campaign effectiveness.
Healthcare
Data analytics in healthcare is used for predicting disease outbreaks, improving patient care, and optimizing operational efficiency.
Finance
In finance, data analytics helps in risk management, fraud detection, and investment strategy formulation.
Retail
Retailers use data analytics to optimize inventory, personalize customer experiences, and improve supply chain efficiency.
Benefits of Data Analytics
Improved Decision Making
Data analytics provides actionable insights that support better decision-making processes.
Increased Efficiency
By analyzing operational data, organizations can identify inefficiencies and streamline processes.
Enhanced Customer Experience
Data analytics helps in understanding customer needs and preferences, leading to improved customer satisfaction.
Competitive Advantage
Organizations leveraging data analytics can stay ahead of competitors by identifying market trends and opportunities.
Challenges in Data Analytics
Data Quality
Ensuring high-quality data is a major challenge in data analytics. Inaccurate or incomplete data can lead to misleading insights.
Data Security
Protecting sensitive data from breaches and unauthorized access is crucial for maintaining data integrity.
Skill Gap
There is a significant skill gap in the field of data analytics. Organizations need skilled professionals to effectively analyze and interpret data.
Data Integration
Integrating data from various sources can be complex and time-consuming.
Latest Innovations in Data Analytics
Artificial Intelligence
AI is revolutionizing data analytics by enabling advanced predictive and prescriptive analytics.
Big Data
Big data technologies allow organizations to analyze vast amounts of data quickly and efficiently.
Cloud Computing
Cloud computing provides scalable and cost-effective solutions for data storage and processing.
Internet of Things (IoT)
IoT devices generate massive amounts of data that can be analyzed for actionable insights.
Future Prospects of Data Analytics
Real-Time Analytics
Real-time analytics will become more prevalent, allowing organizations to make instantaneous data-driven decisions.
Enhanced Personalization
Advancements in data analytics will enable more personalized customer experiences.
Increased Automation
Automation of data analytics processes will reduce manual effort and improve efficiency.
Ethical Data Usage
There will be a greater emphasis on ethical data usage and privacy protection.
Comparative Analysis: Data Analytics vs. Data Science
Definitions
Data analytics focuses on analyzing existing data to draw conclusions, while data science encompasses a broader range of techniques for processing and analyzing data, including machine learning and AI.
Skill Sets
Data analysts typically need skills in statistics and data visualization, while data scientists require advanced programming and machine learning skills.
Applications
Data analytics is used for descriptive and diagnostic analysis, whereas data science is used for predictive and prescriptive analysis.
User Guides: Getting Started with Data Analytics
Step 1: Define Your Objectives
Clearly define what you want to achieve with data analytics. This will guide your data collection and analysis efforts.
Step 2: Collect Relevant Data
Gather data from various sources that are relevant to your objectives.
Step 3: Clean and Prepare Data
Ensure your data is accurate and in a format suitable for analysis.
Step 4: Choose the Right Tools
Select tools and software that best meet your analysis needs.
Step 5: Analyze the Data
Apply appropriate techniques to analyze the data and draw insights.
Step 6: Visualize the Results
Use data visualization tools to present your findings in an easily understandable format.
Step 7: Make Data-Driven Decisions
Use the insights gained from your analysis to inform your decisions and strategies.
Conclusion:
Data analytics is a transformative tool that empowers organizations to make informed decisions, enhance efficiency, and stay competitive in today's data-driven world. By understanding and utilizing the various tools and techniques outlined in this article, beginners can embark on their data analytics journey with confidence. For those seeking to deepen their expertise, enrolling in a Data Analytics Training Institute in Nashik, Ahmedabad, Delhi and other cities in India can provide comprehensive training and hands-on experience to master this essential skill.
Whether you're looking to improve business operations, enhance customer experiences, or unlock new opportunities, data analytics offers the insights needed to achieve your goals. As the demand for data analytics professionals continues to grow, gaining proficiency in this field can open up numerous career prospects and drive innovation across various industries.
Comments