Navigating the Data Deluge: Strategies for Effective Data Analysis
- raginijhaq121
- Mar 18, 2024
- 6 min read
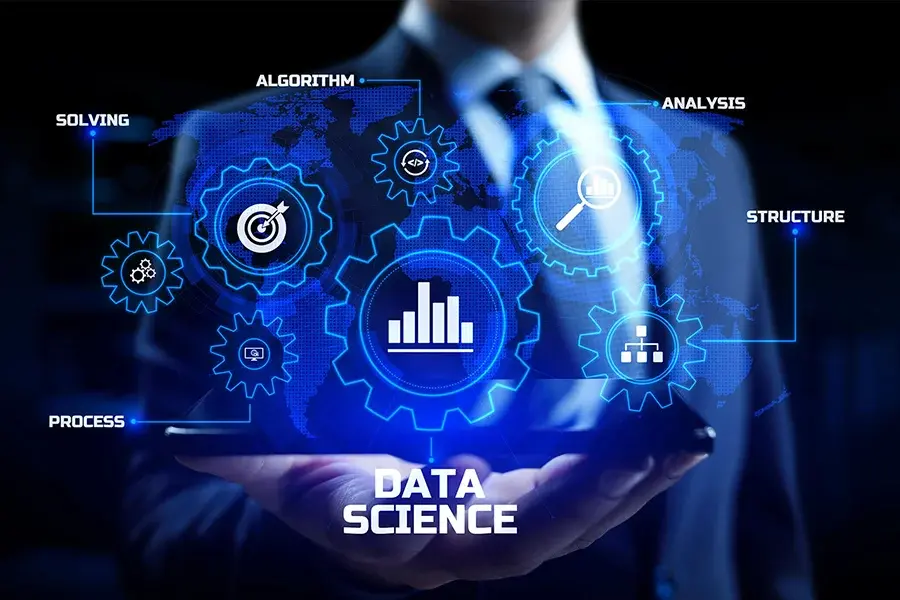
The amount of data that is being produced in the modern digital era is unparalleled. Every click, swipe, and online transaction—from social media conversations to purchases—generates enormous volumes of data. In order to extract relevant insights to support decision-making, organizations are faced with both opportunities and constraints due to the volume of information available. Effective data analysis methods and strategic approaches are needed to navigate this data tsunami.
Understanding the Data Landscape
Before diving into data analysis, it's essential to comprehend the data landscape. Data comes in various forms, including structured, unstructured, and semi-structured data. Structured data, organized in predefined formats like databases, is relatively straightforward to analyze. Unstructured data, such as text, images, and videos, poses challenges due to its lack of predefined structure. Semi-structured data falls somewhere in between, offering a blend of organization and flexibility.
Defining Objectives and Key Metrics
Clarity of objectives is crucial for effective data analysis. Organizations must identify the specific questions they seek to answer or the problems they aim to solve. Whether it's improving operational efficiency, enhancing customer experience, or optimizing marketing campaigns, clear objectives provide direction for the analysis process. Additionally, defining key metrics ensures alignment with business goals and facilitates the measurement of success.
Data Collection and Preparation
The quality of insights derived from data analysis hinges on the quality of the underlying data. Data collection involves gathering relevant information from various sources, such as internal databases, third-party providers, or IoT devices. However, raw data often requires preprocessing and cleansing to address issues like missing values, inconsistencies, and outliers. Data preparation tasks such as cleaning, transformation, and normalization lay the groundwork for accurate analysis.
Selecting Appropriate Analytical Techniques
With data prepared for analysis, the next step is to select suitable analytical techniques. The choice of methods depends on the nature of the data and the objectives of the analysis. Descriptive analytics provides insights into historical data patterns and trends, while predictive analytics forecasts future outcomes based on historical patterns. Meanwhile, prescriptive analytics recommends actions to optimize outcomes based on predictive insights.
Utilizing Advanced Tools and Technologies
Advancements in technology have democratized data analysis, empowering organizations of all sizes to leverage sophisticated tools and platforms. From traditional statistical software like R and Python to modern business intelligence (BI) and data visualization tools, a plethora of options are available to streamline the analysis process. Additionally, cloud computing and big data technologies enable scalable and cost-effective storage and processing of large datasets.
Iterative Analysis and Continuous Improvement
Data analysis is not a one-time endeavor but an iterative process. Continuous monitoring and analysis of key metrics allow organizations to track performance, identify emerging trends, and adapt strategies accordingly. Moreover, feedback loops enable learning from past successes and failures, driving continuous improvement in decision-making processes.
Embracing a Data-Driven Culture
To truly leverage the potential of data analysis, organizations must foster a culture that embraces data-driven decision-making at all levels. This entails not only investing in technical capabilities but also promoting data literacy among employees. Training programs and workshops can empower staff members with the skills and knowledge needed to interpret data effectively and derive actionable insights. Furthermore, leadership support and buy-in are essential to prioritize data initiatives and integrate data analytics into organizational processes and strategies.
Overcoming Challenges and Pitfalls
While data analysis offers immense potential, it also presents challenges and pitfalls that organizations must navigate. Common challenges include data security and privacy concerns, ensuring compliance with regulatory requirements, and managing the complexity of integrating disparate data sources. Moreover, biases in data collection and analysis can lead to erroneous conclusions and flawed decision-making. Addressing these challenges requires a proactive approach, involving collaboration across departments, robust governance frameworks, and ethical guidelines for data use.
Case Studies: Real-World Applications
To illustrate the impact of effective data analysis, consider the following case studies:
Retail Sector: A retail chain leverages customer transaction data and demographic information to personalize marketing campaigns and improve customer retention rates.
Healthcare Industry: A healthcare provider utilizes predictive analytics to forecast patient admission rates, optimize resource allocation, and enhance operational efficiency.
Financial Services: A bank employs machine learning algorithms to detect fraudulent activities, reducing financial losses and enhancing security measures.
These examples highlight how data analysis can drive tangible outcomes and deliver value across diverse industries and domains.
Looking Ahead: Emerging Trends and Opportunities
As technology continues to evolve, new trends and opportunities are reshaping the landscape of data analytics. Artificial intelligence (AI) and machine learning (ML) are unlocking new possibilities for predictive modeling and automation. Meanwhile, the Internet of Things (IoT) is generating vast streams of sensor data, offering insights into real-time operations and consumer behavior. Additionally, advancements in data visualization techniques are making complex information more accessible and actionable for decision-makers.
Embracing Ethical Considerations
Amidst the excitement of data analysis, it's crucial not to overlook ethical considerations. The responsible use of data involves safeguarding privacy, ensuring transparency in data collection and usage, and mitigating biases that may perpetuate inequality or discrimination. Ethical guidelines and frameworks, such as those outlined by regulatory bodies and industry associations, provide essential guidance for ethical data practices. By prioritizing ethics in data analysis, organizations can build trust with stakeholders and mitigate risks associated with data misuse.
Collaborative Innovation and Knowledge Sharing
In the rapidly evolving field of data analytics, collaboration and knowledge sharing are essential for staying abreast of emerging trends and best practices. Industry forums, conferences, and online communities provide platforms for networking and sharing insights with peers and experts. Additionally, partnerships with academic institutions and research organizations foster innovation and drive advancements in data analysis techniques and technologies. By fostering a culture of collaboration and continuous learning, organizations can stay ahead of the curve and unlock new opportunities for growth and innovation.
Final Thoughts: Empowering Data-Driven Decision-Making
In an era defined by data abundance, effective data analysis is no longer a luxury but a necessity for organizations seeking to thrive in a competitive landscape. By adopting strategic approaches, leveraging advanced tools and technologies, and embracing ethical considerations, organizations can navigate the data deluge with confidence and unlock the full potential of their data assets. As data continues to shape the future of business and society, the ability to harness its insights will be a transformative force, driving innovation, efficiency, and sustainable growth in the years to come.
References:
Smith, James (2022). "The Data-Driven Organization: Strategies for Success in the Digital Age." Collins & Harper.
L. Jones and others (2023). "Ethical Guidelines for Data Analytics: Principles and Best Practices." 145–162 in Journal of Data Ethics, 10(2).
R. Patel (2024). "Emerging Trends in Data Analytics: A Comprehensive Review." Computer Science: An International Journal of Advanced Research, 8(1), 78-91.
These references provide valuable insights into the principles, practices, and emerging trends in data analytics, serving as a foundation for organizations embarking on their data-driven journey.
Implementing Agile Methodologies in Data Analysis
In recent years, the application of agile methodologies in data analysis has gained traction, offering a flexible and iterative approach to managing data projects. Borrowing principles from agile software development, such as iterative development, collaboration, and adaptability, agile data analysis promotes faster delivery of insights and greater responsiveness to evolving business needs. By breaking down complex analyses into smaller, manageable tasks and incorporating feedback loops, organizations can enhance the efficiency and effectiveness of their data analysis processes.
Leveraging Data Storytelling for Impactful Communication
Effective communication of data insights is essential for driving informed decision-making and influencing organizational outcomes. Data storytelling, a technique that combines data analysis with narrative elements, helps convey complex information in a compelling and accessible manner. By crafting narratives that contextualize data findings, highlight key insights, and visualize trends and patterns, data storytellers can engage stakeholders, inspire action, and drive meaningful change within organizations.
Investing in Continuous Learning and Development
The field of data analytics is dynamic and constantly evolving, requiring professionals to stay abreast of emerging technologies, methodologies, and best practices. Investing in continuous learning and development initiatives, such as training programs, certifications, and mentorship opportunities, equips individuals with the skills and knowledge needed to excel in their roles and drive innovation in data analysis. By fostering a culture of lifelong learning, organizations can cultivate a highly skilled workforce capable of harnessing the full potential of data to achieve strategic objectives.
conclusion
In conclusion, the evolving landscape of data analytics presents both challenges and opportunities for organizations, highlighting the importance of strategic approaches and continuous learning. As businesses in Delhi and beyond seek to navigate the data deluge, investing in a Data Analytics course in Delhi can equip professionals with the skills and knowledge needed to thrive in this dynamic field. By leveraging advanced methodologies, embracing ethical considerations, and fostering collaboration and innovation, individuals and organizations can harness the transformative power of data to drive informed decision-making, enhance competitiveness, and create value for stakeholders.
Kommentare