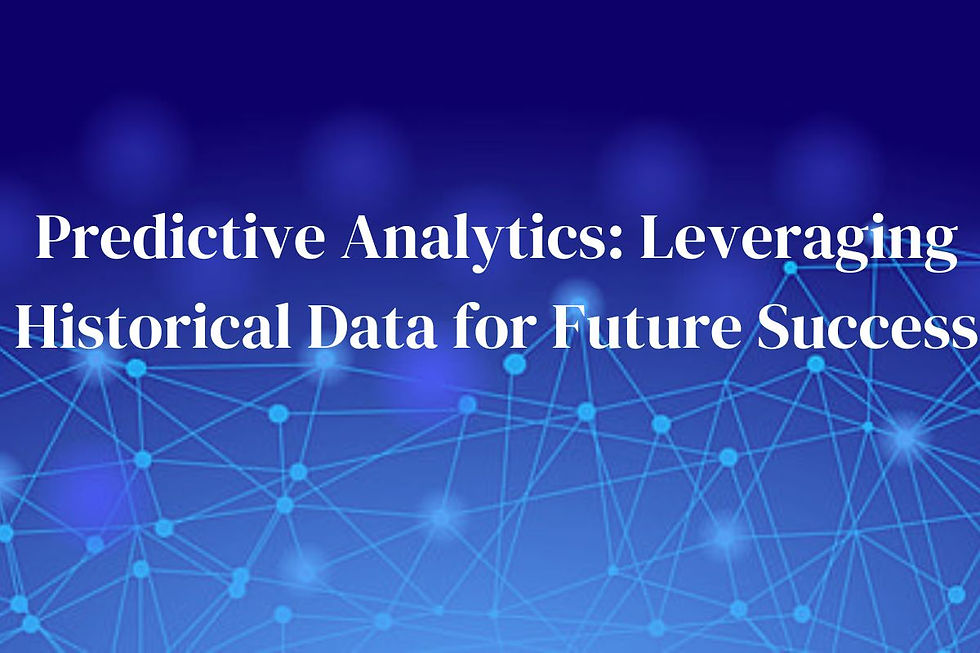
Introduction
Predictive analytics is a transformative technology that leverages historical data to forecast future outcomes. It has become a crucial component in the strategic arsenal of businesses, enabling them to make informed decisions, optimize operations, and enhance customer experiences. This article delves into the evolution, core concepts, applications, benefits, challenges, tools, and future trends of predictive analytics. Additionally, it provides expert insights, case studies, and practical advice for implementing predictive analytics in your business.
The Evolution of Predictive Analytics
Predictive analytics has come a long way from its early days. Initially, it involved simple statistical methods and data analysis techniques. Over time, advancements in technology have significantly enhanced its capabilities.
Historical Background: The roots of predictive analytics can be traced back to early statistical methods developed in the 19th and 20th centuries. These methods laid the foundation for modern predictive models.
Technological Advancements: The advent of computers and the internet revolutionized predictive analytics. The development of sophisticated algorithms and machine learning techniques has further propelled the field.
Key Milestones: Significant milestones in the evolution of predictive analytics include the introduction of decision trees, neural networks, and deep learning models. Each of these advancements has expanded the possibilities of predictive analytics.
Core Concepts of Predictive Analytics
Understanding the core concepts of predictive analytics is essential for leveraging its full potential.
Data Mining: Data mining involves extracting useful information from large datasets. It is the foundation of predictive analytics, enabling the identification of patterns and relationships.
Machine Learning: Machine learning algorithms are at the heart of predictive analytics. They allow systems to learn from data and make predictions without being explicitly programmed.
Statistical Analysis: Statistical techniques are used to analyze data and validate predictive models. They provide a framework for making informed decisions based on data.
Predictive Modeling: Predictive modeling involves creating models that can forecast future outcomes based on historical data. These models are used to predict everything from customer behavior to equipment failures.
Types of Predictive Analytics
Predictive analytics encompasses various types of analyses, each serving a specific purpose.
Descriptive Analytics: Descriptive analytics focuses on summarizing historical data to understand what has happened in the past. It provides a baseline for further analysis.
Diagnostic Analytics: Diagnostic analytics delves deeper into historical data to determine why certain events occurred. It helps identify patterns and root causes.
Predictive Analytics: Predictive analytics uses historical data and machine learning models to forecast future events. It is the primary focus of this article.
Prescriptive Analytics: Prescriptive analytics goes a step further by recommending actions based on predictive insights. It helps organizations optimize their strategies.
Applications of Predictive Analytics
Predictive analytics has diverse applications across various industries.
Marketing: In marketing, predictive analytics is used to understand customer behavior, segment audiences, and personalize campaigns. It helps businesses increase conversion rates and customer loyalty.
Finance: The finance industry leverages predictive analytics for risk management, fraud detection, and investment strategies. It enables more accurate financial forecasting and decision-making.
Healthcare: Predictive analytics is transforming healthcare by enabling early disease detection, personalized treatment plans, and efficient resource allocation. It improves patient outcomes and reduces costs.
Supply Chain Management: In supply chain management, predictive analytics is used to forecast demand, optimize inventory, and prevent disruptions. It enhances operational efficiency and reduces costs.
Human Resources: Predictive analytics helps HR departments with talent acquisition, employee retention, and performance management. It enables data-driven decisions to improve workforce productivity.
Benefits of Predictive Analytics
The benefits of predictive analytics are manifold, offering significant advantages to organizations.
Improved Decision Making: Predictive analytics provides actionable insights that help organizations make informed decisions. It reduces uncertainty and enhances strategic planning.
Increased Operational Efficiency: By predicting demand and optimizing processes, predictive analytics improves operational efficiency. It helps organizations allocate resources effectively.
Enhanced Customer Experience: Predictive analytics enables personalized customer experiences by anticipating customer needs and preferences. It enhances satisfaction and loyalty.
Risk Management: Predictive analytics helps identify potential risks and develop mitigation strategies. It improves the ability to manage uncertainties and reduce adverse impacts.
Challenges in Predictive Analytics
Despite its benefits, predictive analytics faces several challenges.
Data Quality Issues: Poor data quality can significantly impact the accuracy of predictive models. Ensuring data cleanliness and consistency is crucial.
Integration with Existing Systems: Integrating predictive analytics with existing systems can be complex and require significant resources. It is essential to have a seamless integration plan.
Skills Gap: There is a shortage of skilled professionals in predictive analytics. Organizations need to invest in training and development to bridge this gap.
Ethical Considerations: The use of predictive analytics raises ethical concerns, such as data privacy and algorithmic bias. It is important to address these issues proactively.
Tools and Technologies in Predictive Analytics
Various tools and technologies support the implementation of predictive analytics.
Software Solutions: There are numerous software solutions available for predictive analytics, including SAS, IBM SPSS, and RapidMiner. These tools offer a range of features for data analysis and modeling.
Data Warehousing: Data warehousing technologies, such as Amazon Redshift and Google BigQuery, enable organizations to store and manage large datasets for analysis.
Big Data Technologies: Big data technologies, such as Hadoop and Spark, allow organizations to process and analyze vast amounts of data quickly.
Cloud Computing: Cloud platforms, such as AWS, Azure, and Google Cloud, provide scalable infrastructure for predictive analytics. They offer flexibility and cost-effectiveness.
Case Studies
Examining case studies provides insights into the practical applications of predictive analytics.
Successful Implementations: Companies like Amazon and Netflix have successfully implemented predictive analytics to enhance customer experiences and optimize operations.
Lessons Learned: Lessons from these implementations highlight the importance of data quality, skilled personnel, and continuous model evaluation.
Industry-Specific Examples: Predictive analytics is used in various industries, from retail and finance to healthcare and manufacturing, each with unique applications and benefits.
Future Trends in Predictive Analytics
Predictive analytics continues to evolve, with several emerging trends shaping its future.
AI Integration: The integration of artificial intelligence with predictive analytics enhances model accuracy and enables real-time predictions.
Real-Time Analytics: Real-time analytics allows organizations to make immediate decisions based on current data. It is becoming increasingly important in fast-paced industries.
Predictive Maintenance: Predictive maintenance uses predictive analytics to forecast equipment failures and schedule maintenance proactively, reducing downtime and costs.
Enhanced Personalization: Advances in predictive analytics enable more precise personalization, improving customer experiences and driving business growth.
Expert Insights
Gaining insights from industry experts provides valuable perspectives on predictive analytics.
Quotes from Industry Leaders: Industry leaders emphasize the transformative potential of predictive analytics and its role in driving innovation.
Professional Advice: Experts advise organizations to invest in data quality, skilled personnel, and continuous model evaluation to maximize the benefits of predictive analytics.
Future Outlook: The future of predictive analytics is promising, with continued advancements in AI and machine learning expected to drive further innovation.
Implementing Predictive Analytics in Your Business
For organizations looking to implement predictive analytics, there are several key steps to follow.
Steps to Get Started: Start with a clear strategy, define objectives, and identify the necessary data. Choose the right tools and build a skilled team.
Best Practices: Focus on data quality, integrate predictive analytics with existing systems, and continuously evaluate models to ensure accuracy.
Common Pitfalls to Avoid: Avoid common pitfalls such as poor data quality, lack of skilled personnel, and inadequate model evaluation. Ensure ethical considerations are addressed.
Conclusion
Predictive analytics represents a powerful tool for businesses seeking to gain a competitive edge in today's data-driven world. By leveraging historical data and advanced analytical techniques, organizations can anticipate future trends, optimize operations, and enhance decision-making processes. While predictive analytics offers numerous benefits, it is essential for businesses, including data science course providers in Ahmedabad, Nashik, Gurgaon, Delhi and other cities in India to address challenges such as data quality, integration complexities, and ethical considerations to maximize its potential. As the field continues to evolve with advancements in AI and machine learning, staying informed and adopting best practices will be crucial for harnessing the full potential of predictive analytics.
コメント