The Intersection of Data Science and AI: Applications Transformative ns and Opportunities
- raginijhaq121
- Jun 2, 2024
- 5 min read
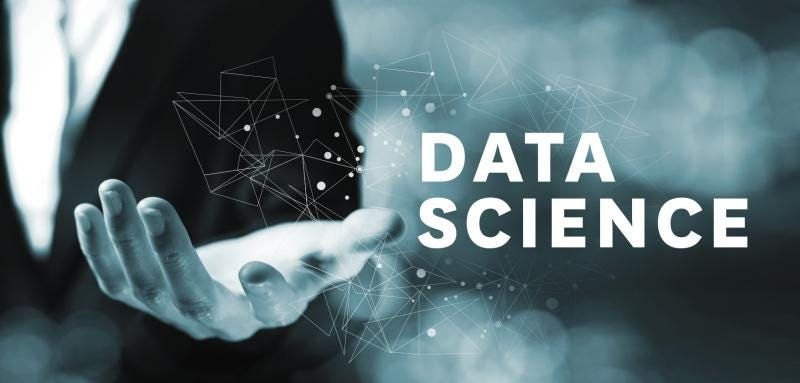
Introduction
Overview of Data Science and AI
Data science and artificial intelligence (AI) are two of the most transformative technologies shaping our world today. Data science involves extracting insights from vast amounts of data using various techniques, while AI refers to the simulation of human intelligence in machines. Together, they form a powerful combination that drives innovation and efficiency across multiple industries.
Historical Development
The origins of data science and AI can be traced back to the early days of computing. Data science evolved from statistics and data analysis, gaining momentum with the advent of big data in the 21st century. AI's development began with the conceptualization of intelligent machines in the mid-20th century, leading to the creation of algorithms that can learn and adapt.
Importance and Relevance Today
In today's digital age, data science and AI are more relevant than ever. They are essential for making sense of the vast amounts of data generated daily, enabling businesses to make informed decisions and offering new opportunities for innovation. Their applications range from healthcare and finance to retail and transportation, impacting every aspect of our lives.
Technical Specifications
Core Concepts of Data Science
Data science encompasses various fields such as statistics, machine learning, and data mining. Key concepts include data collection, cleaning, analysis, and visualization. Techniques like regression analysis, clustering, and classification are fundamental to extracting meaningful insights from data.
Core Concepts of AI
AI involves creating systems capable of performing tasks that typically require human intelligence. This includes machine learning, where algorithms learn from data, and deep learning, which uses neural networks to mimic the human brain. Key concepts include supervised and unsupervised learning, natural language processing, and computer vision.
Data Science and AI Integration
Integrating data science and AI involves combining data-driven insights with intelligent decision-making capabilities. This synergy allows for advanced predictive analytics, real-time data processing, and automated decision-making, significantly enhancing business operations and outcomes.
Tools and Technologies
The integration of data science and AI is facilitated by various tools and technologies. Popular data science tools include Python, R, and SQL, while AI development often utilizes frameworks like Tensor Flow, Py Torch, and Keras. Additionally, cloud platforms such as AWS, Google Cloud, and Azure provide scalable resources for data processing and model training.
Applications
Healthcare
Predictive Analytics
In healthcare, predictive analytics powered by data science and AI can forecast patient outcomes, detect disease outbreaks, and optimize treatment plans. By analyzing historical data, healthcare providers can identify patterns and trends that help in early diagnosis and prevention.
Personalized Medicine
AI-driven personalized medicine tailors treatments to individual patients based on their genetic profiles and medical histories. This approach increases the effectiveness of treatments and reduces adverse effects, leading to better patient outcomes.
Medical Imaging
AI applications in medical imaging include automated image analysis for detecting anomalies such as tumors or fractures. Machine learning algorithms can analyze medical images faster and with greater accuracy than human radiologists, improving diagnostic accuracy and efficiency.
Finance
Fraud Detection
In finance, AI and data science are essential for detecting fraudulent activities. Machine learning models analyze transaction patterns to identify unusual behavior, allowing for real-time fraud detection and prevention.
Algorithmic Trading
Algorithmic trading uses AI to execute trades at optimal times, based on data-driven insights. These algorithms can analyze market trends, predict price movements, and execute trades faster than human traders, maximizing profits.
Risk Management
AI enhances risk management by predicting potential risks and suggesting mitigation strategies. Data science techniques help in assessing credit risk, market risk, and operational risk, enabling financial institutions to make informed decisions.
Retail
Customer Insights
In retail, data science and AI provide deep insights into customer behavior. Analyzing purchase patterns and preferences allows retailers to offer personalized recommendations and improve customer satisfaction.
Inventory Management
AI-driven inventory management systems predict demand, optimize stock levels, and reduce wastage. This ensures that products are available when needed, improving operational efficiency and customer satisfaction.
Personalized Marketing
Personalized marketing powered by AI targets customers with relevant offers and promotions based on their browsing and purchase history. This increases engagement and conversion rates, driving sales growth.
Manufacturing
Predictive Maintenance
In manufacturing, predictive maintenance uses AI to predict equipment failures before they occur. This minimizes downtime, reduces maintenance costs, and extends the lifespan of machinery.
Quality Control
AI-driven quality control systems inspect products for defects with high accuracy. Machine learning algorithms can detect anomalies that might be missed by human inspectors, ensuring higher product quality.
Supply Chain Optimization
AI optimizes supply chains by predicting demand, managing inventory, and improving logistics. This leads to cost savings, faster delivery times, and increased customer satisfaction.
Transportation
Autonomous Vehicles
AI is at the core of autonomous vehicle technology, enabling self-driving cars to navigate safely. Machine learning algorithms process data from sensors and cameras to make real-time driving decisions.
Route Optimization
Route optimization algorithms use AI to determine the most efficient routes for transportation, reducing travel time and fuel consumption. This is beneficial for logistics companies and ride-sharing services.
Traffic Management
AI-based traffic management systems analyze real-time traffic data to optimize traffic flow and reduce congestion. This leads to shorter commute times and lower emissions.
Entertainment
Content Recommendation
Streaming services use AI to recommend content based on user preferences. Machine learning algorithms analyze viewing habits to suggest movies, TV shows, and music that users are likely to enjoy.
Game Development
AI enhances game development by creating realistic environments, characters, and scenarios. It also enables adaptive gameplay, where the game responds to the player's actions in real-time.
Virtual Reality
AI-driven virtual reality (VR) applications provide immersive experiences in gaming, education, and training. Machine learning algorithms create realistic simulations that adapt to user interactions.
Benefits
Enhanced Decision Making
AI and data science provide valuable insights that enhance decision-making processes. By analyzing large datasets, businesses can make informed decisions that improve operational efficiency and drive growth.
Increased Efficiency and Productivity
Automating routine tasks with AI increases efficiency and productivity. Machine learning algorithms can perform complex analyses and repetitive tasks faster than humans, freeing up resources for more strategic activities.
Cost Savings
AI-driven automation and data-driven insights lead to significant cost savings. Predictive maintenance, optimized supply chains, and efficient inventory management reduce operational costs and improve profitability.
Innovation and Competitive Advantage
Adopting AI and data science technologies fosters innovation and provides a competitive advantage. Businesses that leverage these technologies can develop new products and services, improve customer experiences, and stay ahead of competitors.
Challenges and Limitations
Data Privacy and Security
One of the major challenges in AI and data science is ensuring data privacy and security. Protecting sensitive information and complying with data protection regulations are crucial to maintaining trust and avoiding legal issues.
Bias and Fairness
AI algorithms can exhibit bias if trained on biased data, leading to unfair outcomes. Ensuring fairness and avoiding discrimination requires careful data selection and algorithmic transparency.
Technical Complexity
Implementing AI and data science solutions involves technical complexity, requiring specialized skills and knowledge. The need for continuous learning and adaptation to new technologies adds to the challenge.
Ethical Considerations
The use of AI raises ethical concerns, such as the potential for job displacement and the impact on human decision-making. Addressing these ethical considerations is essential to ensure responsible AI development and deployment.
Latest Innovations
Advances in Machine Learning
Recent advances in machine learning have led to the development of more accurate and efficient algorithms. Techniques such as reinforcement learning and transfer learning are pushing the boundaries of what AI can achieve.
Conclusion:
The intersection of data science and AI is revolutionizing industries by providing transformative applications and opportunities. From healthcare and finance to retail and transportation, these technologies enhance decision-making, increase efficiency, and drive innovation. Despite challenges like data privacy and ethical considerations, the benefits far outweigh the drawbacks, making data science and AI indispensable in the modern world.
For those looking to dive deeper into this field, enrolling in a Data Science course in Ahmedabad, Nashik, Noida, Delhi and other cities in India can provide the necessary skills and knowledge to harness these powerful technologies. As advancements continue, staying updated through comprehensive courses will ensure that professionals remain at the forefront of this dynamic landscape.
Comments