The Power of Big Data: Transforming Industries Through Analytics
- raginijhaq121
- May 20, 2024
- 5 min read
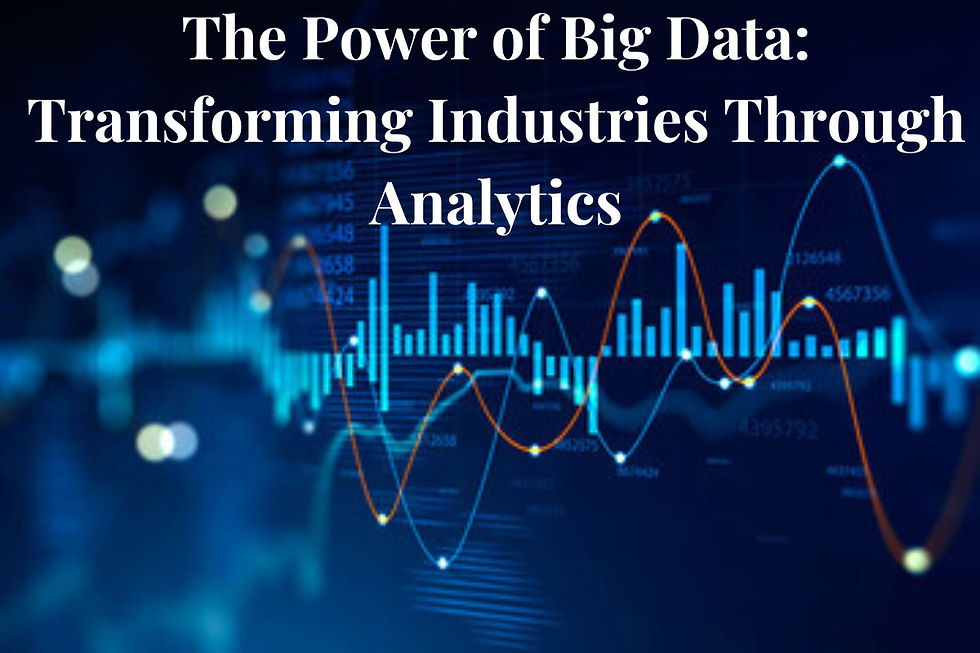
Introduction
Definition of Big Data
Big Data refers to extremely large datasets that are complex and grow at an exponential rate. These datasets are so vast that traditional data processing software cannot manage them efficiently. Big Data encompasses the three Vs: Volume (amount of data), Velocity (speed of data processing), and Variety (types of data).
Evolution and Historical Context
The concept of Big Data is not new; it has evolved alongside advancements in technology. In the 1960s, data processing involved simple databases and mainframe computers. The 1980s saw the introduction of relational databases and data warehousing. With the advent of the internet in the 1990s, data generation surged, leading to the current era of Big Data characterized by the use of sophisticated analytics to derive insights from diverse and vast datasets.
Importance and Relevance in Modern Industries
In today's digital age, data is a critical asset for organizations across all sectors. The ability to analyze and leverage data can lead to significant competitive advantages, driving decision-making, enhancing customer experiences, and optimizing operations. Big Data analytics helps organizations to uncover hidden patterns, correlations, and insights, enabling more informed business strategies.
Overview of Big Data Analytics
Big Data Analytics involves the use of advanced analytics techniques on large, diverse datasets that include structured, semi-structured, and unstructured data, from different sources and in different sizes. Techniques such as machine learning, data mining, predictive analytics, and natural language processing (NLP) are employed to extract valuable insights.
Types and Categories of Big Data
Structured Data
Structured Data is highly organized and easily searchable by simple, straightforward search engine algorithms or other search operations. This includes data stored in databases, spreadsheets, and SQL databases, such as names, dates, and addresses.
Unstructured Data
Unstructured Data lacks a predefined format or structure, making it more challenging to collect, process, and analyze. Examples include text files, emails, social media posts, and multimedia files like images and videos.
Semi-structured Data
Semi-structured Data does not conform to the formal structure of data models but contains tags or markers to separate data elements. Examples include XML, JSON files, and NoSQL databases.
Metadata
Metadata is data that provides information about other data. Examples include author, date created, date modified, and file size. Metadata is crucial for data management and retrieval.
Real-time Data
Real-time Data is information that is delivered immediately after collection. There is no delay in the timeliness of the information provided. Examples include stock market feeds, social media updates, and real-time sensors in smart devices.
Big Data Technologies
Hadoop Ecosystem
Hadoop is an open-source framework that allows for the distributed processing of large datasets across clusters of computers using simple programming models. Components include Hadoop Distributed File System (HDFS), MapReduce, Hadoop Common, and Hadoop YARN.
Apache Spark
Apache Spark is a unified analytics engine for large-scale data processing, with built-in modules for streaming, SQL, machine learning, and graph processing. It provides high-level APIs in Java, Scala, Python, and R, and an optimized engine that supports general execution graphs.
NoSQL Databases
NoSQL databases are designed for the storage, retrieval, and management of large volumes of data. Unlike traditional relational databases, NoSQL databases use a variety of data models, including document, key-value, column-family, and graph formats.
Data Warehousing Solutions
Data Warehousing involves the collection of data from different sources into one comprehensive and easily queryable format. Solutions like Amazon Redshift, Google BigQuery, and Snowflake are popular for their scalability and efficiency.
Cloud Computing Platforms
Cloud computing platforms like Amazon Web Services (AWS), Microsoft Azure, and Google Cloud provide scalable resources for storage, computing, and big data analytics. They offer services such as data lakes, AI tools, and managed databases.
Data Lakes
Data Lakes are centralized repositories that allow you to store all your structured and unstructured data at any scale. They enable organizations to run different types of analytics—from dashboards and visualizations to big data processing, real-time analytics, and machine learning.
Applications of Big Data in Various Industries
Healthcare
Predictive Analytics
In healthcare, predictive analytics is used to forecast patient outcomes based on historical data. This can help in predicting disease outbreaks, hospital readmission rates, and the likelihood of certain treatments being effective.
Personalized Medicine
Big Data enables the analysis of genetic information alongside lifestyle and clinical data to tailor personalized treatment plans, improving patient outcomes and reducing costs.
Electronic Health Records
Electronic Health Records (EHRs) are digital versions of patients' paper charts. Big Data analytics helps in managing and analyzing these records for better patient care and operational efficiency.
Finance
Fraud Detection
Financial institutions use Big Data analytics to detect and prevent fraudulent activities by analyzing transaction patterns and identifying anomalies that may indicate fraud.
Risk Management
Big Data helps in assessing risks by analyzing vast amounts of historical data. This is crucial for financial institutions to make informed decisions regarding loans, investments, and insurance.
Customer Segmentation
Big Data analytics allows financial institutions to segment customers based on their behaviors and preferences, enabling targeted marketing and personalized services.
Retail
Customer Experience Optimization
Retailers use Big Data to analyze customer data and improve their shopping experience. This includes personalized recommendations, optimized store layouts, and enhanced customer service.
Inventory Management
Big Data analytics helps retailers in managing inventory by predicting demand and optimizing stock levels, reducing costs and increasing efficiency.
Pricing Strategies
Retailers can use Big Data to develop dynamic pricing strategies based on market trends, competitor pricing, and customer behavior, ensuring competitive pricing and maximizing profits.
Manufacturing
Predictive Maintenance
Manufacturers use Big Data analytics to predict equipment failures before they occur, reducing downtime and maintenance costs while improving productivity.
Supply Chain Optimization
Big Data enables manufacturers to optimize their supply chains by analyzing data from suppliers, production lines, and customers, leading to reduced costs and improved efficiency.
Quality Control
Big Data analytics helps in monitoring production processes and product quality in real-time, identifying defects early, and ensuring high-quality products.
Transportation and Logistics
Route Optimization
Big Data analytics is used to optimize routes for logistics and transportation companies, reducing delivery times and fuel consumption.
Fleet Management
Big Data helps in managing fleets by monitoring vehicle conditions, driver behavior, and fuel usage, leading to improved efficiency and reduced costs.
Traffic Prediction
Analyzing traffic data helps in predicting congestion and optimizing traffic flows, improving transportation efficiency and reducing travel times.
Energy
Smart Grid Management
Big Data analytics is crucial for managing smart grids, optimizing energy distribution, and predicting power consumption patterns to balance supply and demand.
Predictive Maintenance
Energy companies use Big Data to predict and prevent equipment failures, reducing maintenance costs and ensuring reliable energy supply.
Conclusion:
The transformative power of Big Data analytics is reshaping industries across the globe. From healthcare to finance, retail to manufacturing, organizations are leveraging the vast potential of Big Data to drive innovation, enhance operational efficiency, and gain a competitive edge. As technology continues to advance and data generation escalates, the demand for skilled professionals proficient in Big Data analytics is on the rise. For those looking to capitalize on this growing field, enrolling in a Data Analytics course in Ahmedabad, Nashik, Delhi and other cities in India can provide the necessary skills and knowledge to thrive in the digital age. By embracing Big Data analytics and investing in education and training, individuals and organizations alike can unlock new opportunities and navigate the complexities of the data-driven future with confidence.
Comentarios